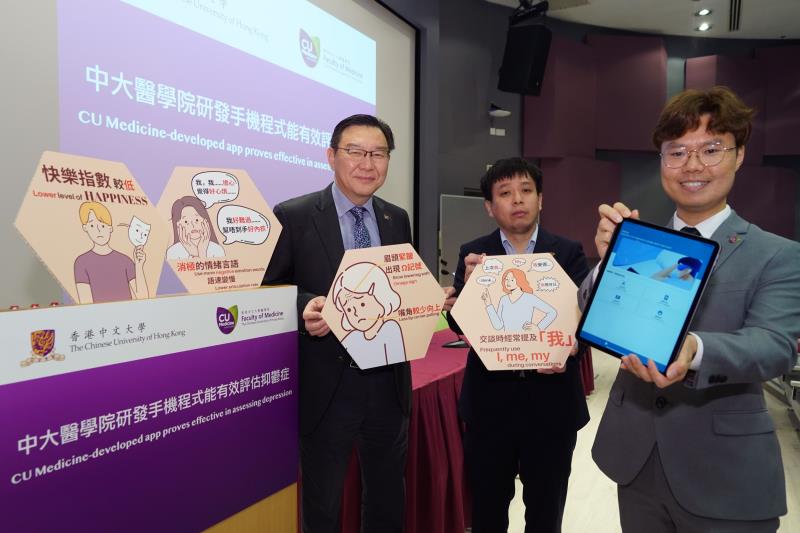
Researchers from the Chinese University of Hong Kong (CUHK) have developed an artificial intelligence (AI)–powered app and actigraphy that effectively diagnoses depression by analyzing rest-activity, facial expression, mood, and voice.
“Depression goes beyond just feeling sad and involves a range of [facial expression,] mood, language, and rest-activity changes,” noted Professor Wing-Yun Kwok of the Department of Psychiatry, CUHK. Therefore, the researchers developed a multimodal digital assessment to aid depression diagnosis. [Transl Psychiatry 2024;14:150]
In a case-control study in Hong Kong, 101 patients with depression and 82 individuals without psychiatric disorders (controls) underwent 1 week of the multimodal AI-powered digital assessment, which included using actigraphy to measure rest-activity patterns and using an app to rate subjective mood state as well as capture facial expressions and voice during description of feelings in video clips. Eighty patients with depression and 76 controls completed the actigraphy, while 61 patients with depression and 47 controls completed the app-based assessment. Results showed that patients with depression demonstrated several features compared with controls:
Facial expression: More frequent brow lowering (p=0.023) and less lip corner pulling (p=0.007) – also known as the omega [Ω] sign
- Rest-activity pattern: Reduced level of physical activity (p=0.006) as well as delayed sleep midpoint (p=0.047) and acrophase (p=0.024)
- Speech: Lower articulation rate (p<0.001), higher pause variability (p=0.046), more self-reference (p=0.018) and negative emotion words (p<0.001)
- Mood states: Lower average subjective happiness level, regardless of remission status (both p<0.05).
“In line with previous Caucasians studies, we observe a decreased rate of articulation and a tendency to use more first-person singular pronouns in the Chinese population, which reflects a universal cross-cultural phenomenon of self-focused attention in patients with depression,” added Wing.
Of note, the multimodal AI-powered digital assessment applied seven machine learning models. “The performance score was satisfactory (F1 score, 0.81) in predicting lifetime diagnosis of depression, which was superior to that of any individual modality including Hospital Anxiety and Depression Scale – depression subscale [HADS-D],” reported Dr Jie Chen of the Department of Psychiatry, CUHK. “The F1 performance score was 0.64 in predicting non-remission status, which was further improved to 0.70 with the addition of HADS-D.”
The prevalence rate of depression in Hong Know was 9.1 percent in a 10-year prospective cohort study, indicating a high demand for mental health services. However, the 2015 Hong Kong Mental Morbidity Survey showed that >70 percent of patients with common mental disorders (eg, depression) had not consulted mental health services, which could be due to a lack of awareness, inaccessibility, and stigma. [https://www.familycohort.sph.hku.hk/home; Soc Psychiatry Psychiatr Epidemiol 2015;50:1379-1988]
The emerging potential of utilizing multimodal AI for digital assessment could offer a continuous, unobtrusive, objective assessment of depression. “The new technology has the potential to assist diagnosis and monitoring of depression, alleviating the burden on healthcare providers,” commented Dr Man-Ho Li of the Department of Psychiatry, CUHK.