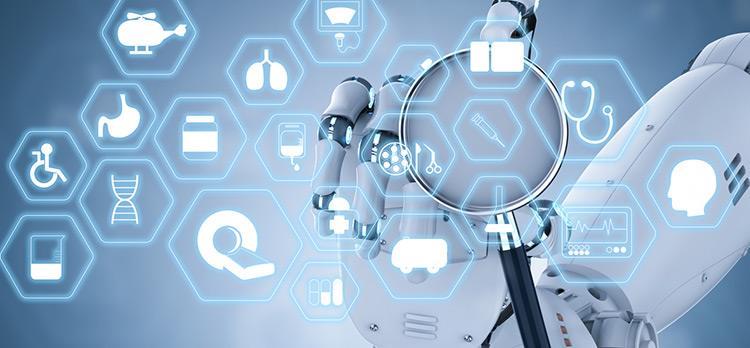
Analysis of routine clinical data via machine learning show promise for predicting the progression to schizophrenia within 5 years among patients with pre-existing mental illness, as shown in a study.
Researchers used data from electronic health records (EHRs) from 24,449 patients between 15 and 60 years with at least two contacts (at least 3 months apart) with the Psychiatric Services of the Central Denmark Region.
The main outcome was diagnostic transition to schizophrenia or bipolar disorder within 5 years, predicted 1 day before outpatient contacts using elastic net regularized logistic regression and extreme gradient boosting (XGBoost) models.
The median age of the cohort at time of prediction was 32.2 years, and more than half (56.6 percent) were women. The XGBoost model was able to predict transition to the first occurrence of either schizophrenia or bipolar disorder, with an area under the receiver operating characteristic curve (AUROC) of 0.70 (95 percent confidence interval [CI], 0.70–0.70) for the training set and 0.64 (95 percent CI, 0.63–0.65) for the test set, which consisted of two held-out hospital sites.
At a predicted positive rate of 4 percent, the XGBoost model achieved a 9.3-percent sensitivity, a 96.3-percent specificity, and a 13-percent positive predictive value (PPV). The model notably performed better for predicting progression to schizophrenia (AUROC, 0.80, 95 percent CI, 0.79–0.81; sensitivity 19.4 percent, specificity 96.3 percent, PPV 10.8 percent) than to bipolar disorder (AUROC, 0.62, 95 percent CI, 0.61–0.63; sensitivity 9.9 percent, specificity 96.2 percent, PPV 8.4 percent). Clinical notes proved particularly informative for prediction.