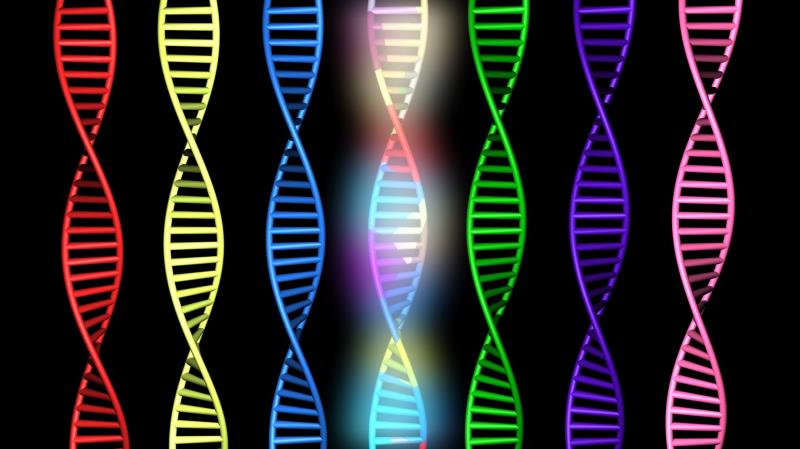
DeepGEM, a novel tool that harnesses artificial intelligence (AI), delivers accurate, timely, and economical gene mutation prediction from routinely acquired histology slides, enhancing the efficiency of lung cancer mutation screening, according to a study.
Developed by a team of China-based researchers, DeepGEM addresses the gap in traditional genomic testing by using whole slide images (WSIs) of aspirational and excisional biopsies. This approach is a step up from next-generation sequencing (NGS), which requires high-quality tissue samples and can be an expensive and time-consuming process, limiting its accessibility to many patients, particularly in low-resource settings, reported first study author Dr Shan Xiong from the China State Key Laboratory of Respiratory Disease & National Clinical Research Center for Respiratory Disease, First Affiliated Hospital of Guangzhou Medical University in Guangzhou, China.
DeepGEM is an annotation-free AI approach that leverages the weekly supervised multiple instance learning, as well as instance-level and bag-level co-supervision to perform gene mutation prediction. Whole slide images are processed and analysed to estimate the probability of common driver gene mutations such as EGFR, KRAS, ALK, ROS1, TP53, and LRP1B.
Xiong highlighted another interesting feature of DeepGEM, which is its ability to generate spatial gene mutation maps showing the distribution of mutations.
Performance training and testing
Xiong and colleagues used datasets from 16 centres, 3,434 patients, and 3,436 WSIs to train and test the performance of DeepGEM. The dataset, which included paired pathological images and gene mutation data, was complemented by publicly available datasets from The Cancer Genome Atlas (multiple centres, 473 patients, 535 WSIs).
DeepGEM was initially trained and evaluated on an internal dataset of 1,717 WSIs and subsequently tested on an external dataset of 1,719 WSIs and a public dataset of 535 WSIs. A dataset of 331 WSIs of lymph node metastases biopsies was also used to evaluate the model’s generalizability.
“DeepGEM outperformed other existing deep learning-based pathological imaging methods in the internal dataset and exhibited a robust performance in the external dataset,” Xiong said.
In the internal dataset, DeepGEM achieved a median area under the curve (AUC) of 0.939 for excisional biopsies, 0.883 for aspiration biopsies, and 0.881 for all biopsies. In the external dataset, the median AUCs were 0.860 for excisional biopsies, 0.853 for aspiration biopsies, and 0.842 for all biopsies. [WCLC 2024, abstract OA03.05]
Xiong noted that the model had a median AUC of 0.872 in the TCGA dataset, indicating adaptability across diverse racial backgrounds. Additionally, the model demonstrated robust generalization ability, with a median AUC of 0.911 for EGFR and 0.882 for KRAS in the lymph node metastases dataset, she added.
When the association between the prediction probability and the survival outcomes were investigated, patients with a high predicted EGFR mutation probability had significantly better survival outcomes (p=0.024).
As for the spatial gene mutation maps generated by DeepGEM, “the high-probability EGFR mutation regions indicated by DeepGEM were consistent with the regions indicated by corresponding immunohistochemistry stains,” Xiong pointed out.
Paving the way for precision treatment
“Rapid and precise detection of driver gene mutations is crucial for precision treatment for lung cancer patients,” according to Xiong.
“The rapid prediction capabilities of DeepGEM allow for quicker decision-making in treatments, enabling patients with severe symptoms to receive targeted therapies sooner,” which is particularly beneficial in economically underdeveloped areas where genomic testing is unaffordable, she said. “DeepGEM presents opportunities for multi-gene detection and precision treatment in these regions.”