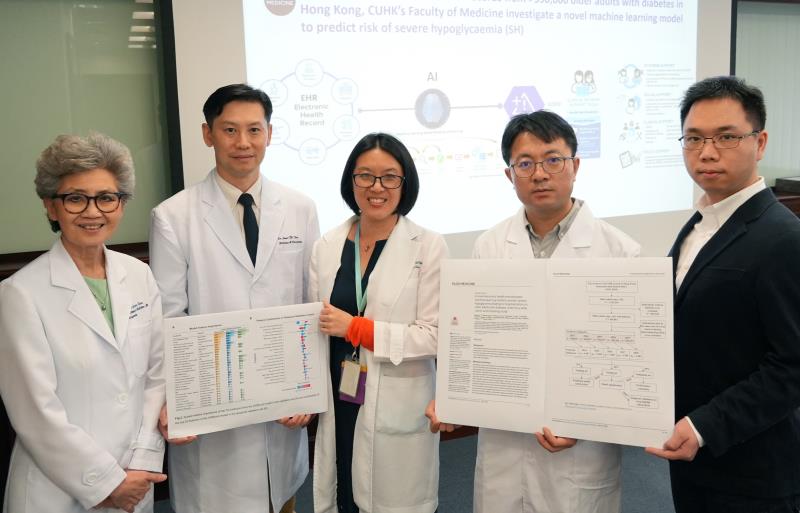
Researchers from the Chinese University of Hong Kong (CUHK) have developed a novel machine learning–based model for predicting 1-year risk of severe hypoglycaemia in older patients with diabetes mellitus (DM).
“Integrating the model with the local electronic health record system allows identification of [DM] patients at high risk of severe hypoglycaemia, facilitating tailored treatment adjustments such as transitioning to therapies with lower hypoglycaemic risk or optimizing insulin therapy,” said lead investigator Dr Elaine Chow of the Department of Medicine and Therapeutics, CUHK.
Severe hypoglycaemia remains a feared complication in older DM patients. [Diabetes Care 2021;44:e40-e41] “In Hong Kong, hospitalization is required for >80 percent of older patients presenting with hypoglycaemia at emergency departments [EDs],” said Dr Jones Chan of the Department of Medicine and Therapeutics, Prince of Wales Hospital.
For early identification of older DM patients at high risk of severe hypoglycaemia, the researchers used a case-control design to develop a machine learning–based model for predicting 1-year risk of severe hypoglycaemia requiring hospitalization. Six machine learning algorithms were employed to train prediction models on randomized subsets of cohort data from the Hospital Authority Data Collaboration Lab (HADCL), which comprised approximately 1.5 million records of about 360,000 DM patients aged ≥65 years who attended HA services in 2013–2018. [PLoS Med 2024;21:e1004369]
The machine learning–based models incorporated 258 select parameters, such as demographics, admissions, diagnoses, medications, and routine laboratory tests over a 1-year period, to predict severe hypoglycaemia events requiring hospitalization in the subsequent 12 months. The conventional risk prediction strategy employed an 11-variable logistic-regression model that comprised risk factors such as age, gender, history of severe hypoglycaemia, hypertension, blood glucose levels, kidney function measurements, and use of oral glucose-lowering drugs.
During the observational period of the study, there were 11,128 severe hypoglycaemia events requiring hospitalization in 9,616 patients. The XGBoost model showed the best performance among evaluated machine learning models, achieving an area under the receiver operating characteristic curve (AUROC) of 0.978, an area under the precision-recall curve (AUPRC) of 0.670 and positive predictive value (PPV) of 0.721. These results were superior to those of the conventional model (AUROC, 0.906; AUPRC, 0.085; PPV, 0.468).
In the XGBoost prediction model, key predictors of severe hypoglycaemia risk included nonuse of lipid-lowering drugs, use of insulin, use of sulfonylureas, number of inpatient records, and urgent triage category during ED attendance. Other parameters, such as outpatient appointment specialty, types of ward care, and residential district, may also contribute to risk of severe hypoglycaemia.
The robustness of the XGBoost-based prediction model was assessed using a temporal validation cohort from the Hong Kong Diabetes Register, where predictors were collected in 2018 and hospitalizations due to severe hypoglycaemia were recorded in 2019. Among 14,295 clinical records from 13,917 DM patients alive by the end of 2019, the XGBoost-based prediction model identified 722 events, resulting in AUROC of 0.856 and AUPRC of 0.286.
“Our machine learning model is highly efficient and cost-effective for identifying older [DM] patients at high risk of hospitalization due to severe hypoglycaemia,” commented Professor Juliana Chan of the Department of Medicine and Therapeutics, CUHK. The researchers plan to evaluate the model’s effectiveness in identifying patients with less severe hypoglycaemia.