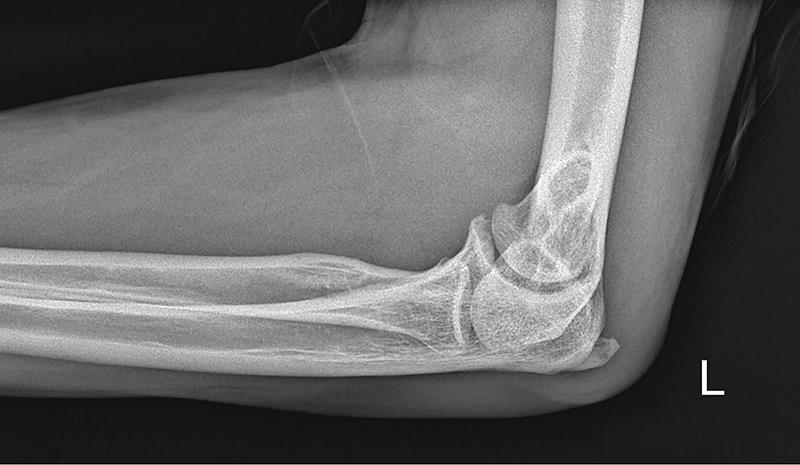
A deep learning artificial intelligence (AI) model demonstrates good area under the receiver operating characteristic curve (AUROC) and higher sensitivity in the binomial classification of paediatric elbow radiographs when compared to emergency department (ED) physicians, reports a Singapore study.
“The initial model developed in this study is planned for further refinement with a view for future deployment within the paediatric and adult hospital setting for image triage into normal and abnormal groups at the point of care by the attending clinician,” the investigators said.
The test set revealed an accuracy of 80.4 percent (95 percent confidence interval [CI], 71.8‒87.3) for the AI model, with an AUROC of 0.872 (95 percent CI, 0.831‒0.947). [Singapore Med J 2025;66:208-214]
When compared with the physician group, the AI model demonstrated a sensitivity of 79.0 percent (95 percent CI, 68.4‒89.5 vs 64.9 percent, 95 percent CI, 52.5‒77.3; p=0.088) and a specificity of 81.8 percent (95 percent CI, 71.6‒92.0 vs 87.3 percent, 95 percent CI, 78.5‒96.1; p=0.439).
“This form of binomial classification models may have a role as a decision support tool for clinicians in the acute care setting and as a decision support algorithm for radiologists, for radiology worklist management, and for identification of potentially abnormal radiographic images for expedited interpretation by a radiologist,” the investigators said. [AJR Am J Roentgenol 2018;211:1361-1368]
Previous models
However, the AI model in this study showed an inferior performance relative to that of previously developed models, which had an accuracy of 88 percent to 91 percent, a sensitivity of 91 percent to 93 percent, and specificity of 84 percent to 92 percent. [Pediatr Radiol 2022;52:2149-2158; Radiol Artif Intell 2019;1:e180015; Investig Radiol 2020;55:101-110]
“The reasons for this are not immediately apparent; possible explanations for the variance may include different cases, set sizes, and set hyperparameters,” the investigators said.
In the study by Rayan and colleagues, researchers used an Xception network architecture and a larger dataset with 58,817 images. The images presented to the model were resized to 500 x 500 resolution. In another study, Choi and colleagues used a ResNet-50 network architecture, and the images were resized to a 600 x 600 resolution.
“Although increased resolution may not always correlate with model performance owing to decreases in maximum batch size, other studies assessing the effect of image resolution … have described improved model performance for detection of small and subtle features with increased image resolution,” the investigators said. [IEEE Trans Neural Netw 1994;5:537-550; Radiol Artif Intell 2020;2:e190015]
The current study included a total of 1,314 paediatric elbow lateral radiographs (patient mean age 8.2 years), retrospectively reviewed and classified based on annotation as normal or abnormal (with pathology). These images were then randomly partitioned to a development set (n=993), first and second tuning sets (validation; n=109 and 100, respectively), and a test set (n=112).
The investigators trained an AI model on the development set using the EfficientNet B1 network architecture. They compared its performance on the test set with that of five physicians (inter-rater agreement: fair). Finally, the McNemar test was used to assess the performance of the AI model and the group of physicians.