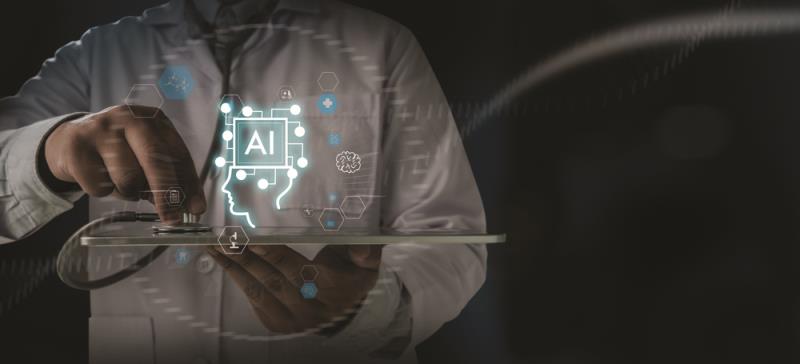
The world’s first artificial intelligence (AI) model for diagnosis of well-differentiated thyroid cancer, developed by researchers from the University of Hong Kong (HKU), has demonstrated high accuracy in staging and risk classification based on analysis of semi-structured free-text clinical notes.
Using pathology reports from 339 patients in The Cancer Genome Atlas Thyroid Cancer (TCGA-THCA) programme’s public data set and clinical notes of 35 pseudo cases, the researchers developed a named entity framework for information extraction and examined large language model (LLM) strategies for staging by the 8th edition of the American Joint Committee on Cancer (AJCC) criteria as well as risk classification by the American Thyroid Association (ATA) criteria. [NPJ Digit Med 2025;8:134]
The training set included 50 TCGA-THCA patients, while the validation set included 289 TCGA-THCA patients and 35 pseudo cases. Stage distribution of the TCGA-THCA cases aligned with population-based epidemiological data, with stages I and II accounting for >90 percent of cases. Clinical notes of the pseudo cases were created and labelled with ground truth by two endocrine surgeons to resemble the format and content of semi-structured clinical notes in Hong Kong, where application of the AI model was intended and real clinical notes were inaccessible for the current study due to data privacy concerns.
Four offline open-source LLMs (ie, Mistral AI’s Mistral-7B-Instruct-v0.3, Google’s Gemma-2-9B-Instruct, Meta’s Llama 3.1-8B-Instruct, and Alibaba’s Qwen2.5-7B-Instruct) were used to extract cancer-related information from semi-structured clinical notes.
By combining the output of all four LLMs, the AI model achieved overall accuracy of 92.9–98.1 percent for AJCC cancer staging and 88.5–100 percent for ATA risk classification.
“A significant advantage of this AI model is its offline capability, which could allow local deployment without the need to share or upload sensitive patient information, thereby preserving patients’ privacy when real clinical notes are used,” said Professor Joseph Wu of HKU’s School of Public Health and InnoHK Laboratory of Data Discovery for Health (InnoHK D24H).
“Further comparative tests with a ‘zero-shot approach’ against the latest versions of DeepSeek – R1 and V3 – as well as GPT-4o showed that our model performed on par with these powerful online LLMs,” Wu added.
“In addition to the high accuracy in extracting and analyzing information from complex pathology reports, operation records and clinical notes, our AI model also nearly halves doctors’ preparation time compared with human interpretation of information,” said Dr Matrix Fung of HKU’s Department of Surgery.
“The AI model is versatile and could be readily integrated into various settings in the public and private sectors, as well as both local and international healthcare and research institutions,” Fung continued. “Real-world implementation of this AI model could enhance the efficiency of frontline clinicians and improve the quality of care, giving doctors more time for patient counselling.”
“In line with the government’s strong advocacy of AI adoption in healthcare, as exemplified by the recent launch of LLM-based medical report writing in the Hospital Authority, our next step is to evaluate the performance of this AI model with a large amount of real-world patient data,” said Dr Carlos Wong of HKU’s Department of Family Medicine and Primary Care.