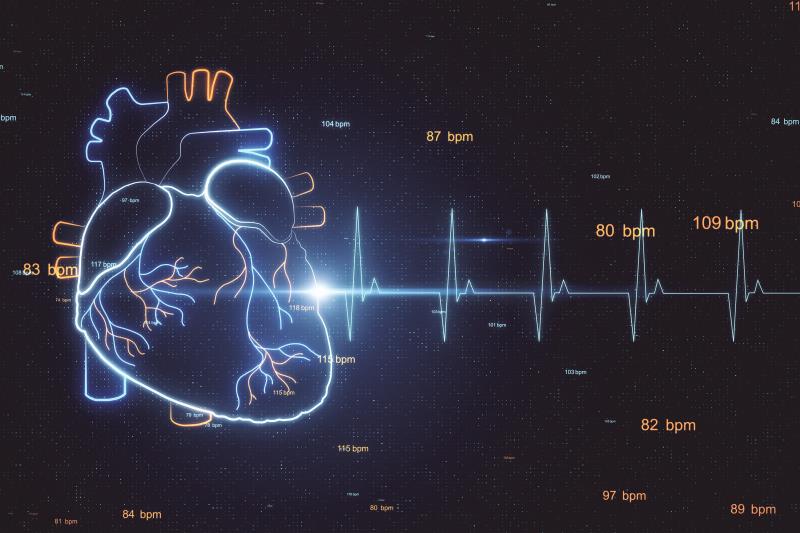
An ECG enhanced by artificial intelligence (AI) demonstrates its capability in detecting biventricular dysfunction and dilation in patients with congenital heart disease (CHD).
A team of investigators trained and tested a convolutional neural network on paired ECG-cardiovascular magnetic resonance (CMR) ≤30 days apart from patients with and without CHD to diagnose left ventricular (LV) dysfunction (ejection fraction ≤40 percent), right ventricular (RV) dysfunction (ejection fraction ≤35 percent), and LV and RV dilation (end-diastolic volume z-score ≥4).
The investigators performed internal testing and external validation on an outside healthcare system to assess performance using area under receiver-operating curve (AUROC) and are under precision recall curve. The internal and external cohorts consisted of 8,584 ECG-CMR pairs (n=4,941; median CMR age 20.7 years) and 909 ECG-CMR pairs (n=746; median CMR age 25.4 years), respectively.
Model performance was comparable for internal (AUROC: LV dysfunction, 0.87; LV dilation, 0.86; RV dysfunction, 0.88; RV dilation, 0.81) and external validation (AUROC: LV dysfunction, 0.89; LV dilation, 0.83; RV dysfunction, 0.82; RV dilation, 0.80), and lowest in functionally single ventricular patients.
Patients with tetralogy of Fallot who were predicted to have a high risk of ventricular dysfunction had poorer survival (p<0.001).
In addition, “[m]odel explainability via saliency mapping revealed that lateral precordial leads influence all outcome predictions, with high-risk features including QRS widening and T-wave inversions for RV dysfunction/dilation,” according to the investigators.