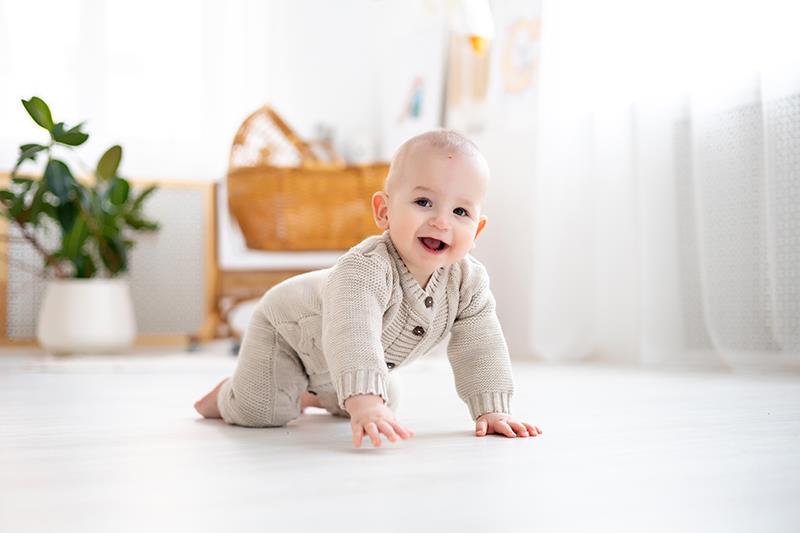
New research has demonstrated the ability of artificial intelligence (AI) algorithms to reliably and automatically assess the gross motor skills of infants from everyday wearable data.
Developed by a team of scientists from the University of Helsinki, Helsinki, Finland, the algorithms were designed to detect the reaching of gross motor milestones, quantify times spent in different postures, and longitudinally track gross motor development.
The gross motor milestone analysis was based on the World Health Organization’s (WHO) multicentre reference study, which provides two benchmarking measures: the age range for achieving the milestones within a large cohort and the expert-validated detection accuracy. The milestones examined included unsupported sitting, crawling on all fours, standing, and walking. Prone crawling or ‘commando crawling’ was also analysed as a measure of early independent movement.
Data used for the analysis were obtained from a smart jumpsuit (MAIJU) that equips four movement sensors, one in each sleeve. Parents dressed their infants in MAIJU and then allowed the infants to play freely for at least 1 hour. The sensors sent the data to a nearby mobile device via a Bluetooth connection, and the data were uploaded to a server for automated analysis.
“This technique brings objectivity and global harmonization to the assessment of motor development. It also boosts regional equality in children’s developmental assessment both nationally and internationally,” said senior scientist Professor Sampsa Vanhatalo from the University of Helsinki.
Algorithm performance
The algorithms’ performance was evaluated using 620 at-home measurements collected from 134 children between 4 and 22 months of age. The measurements taken from 97 infants (47 boys) were used for the algorithm development and cross-validation, whereas those taken from 37 (23 boys) were used for external validation.
The algorithms demonstrated high accuracy for detecting gross motor milestone achievement. Cross-validation accuracy ranged from 90.9 percent to 95.5 percent, while external validation accuracy ranged from 92.4 percent to 96.8 percent. This performance compared well with the inter-rater reliability observed among human experts in the WHO reference study. [Pediatrics 2025;doi:10.1542/peds.2024-068647]
Spearman’s rho correlations indicated strong agreement between wearable-derived postural times and parental assessments (ρ=0.48–0.81), as well as between individual motor maturation trajectories and infant age (ρ=0.93).
Health and research applications
“This is the first study, to our knowledge, to demonstrate that at-home wearable measurements provide objective, reliable, quantified, scalable, and ecologically valid assessments of infants’ gross motor skills,” said Vanhatalo and colleagues. “The measures presented are biologically and behaviourally reasoned, transparent, and intuitively explainable.”
According to the scientists, the approach has diverse health and research applications including early detection of developmental delays in at-risk infants, exploring the connections between gross motor and other developmental delays, facilitating comparative studies across neurodevelopmental conditions, serving as a clinical decision-support tool in well-child care, and providing objective outcome measures for interventional and observational studies.
It can also enable personalized therapy targets and efficacy metrics, inform evidence-based developmental care guidelines, help in the creation of culture- and language-independent infant assessments for international data pooling, and, ultimately, promote equitable infant healthcare through accessible electronic solutions, they said.